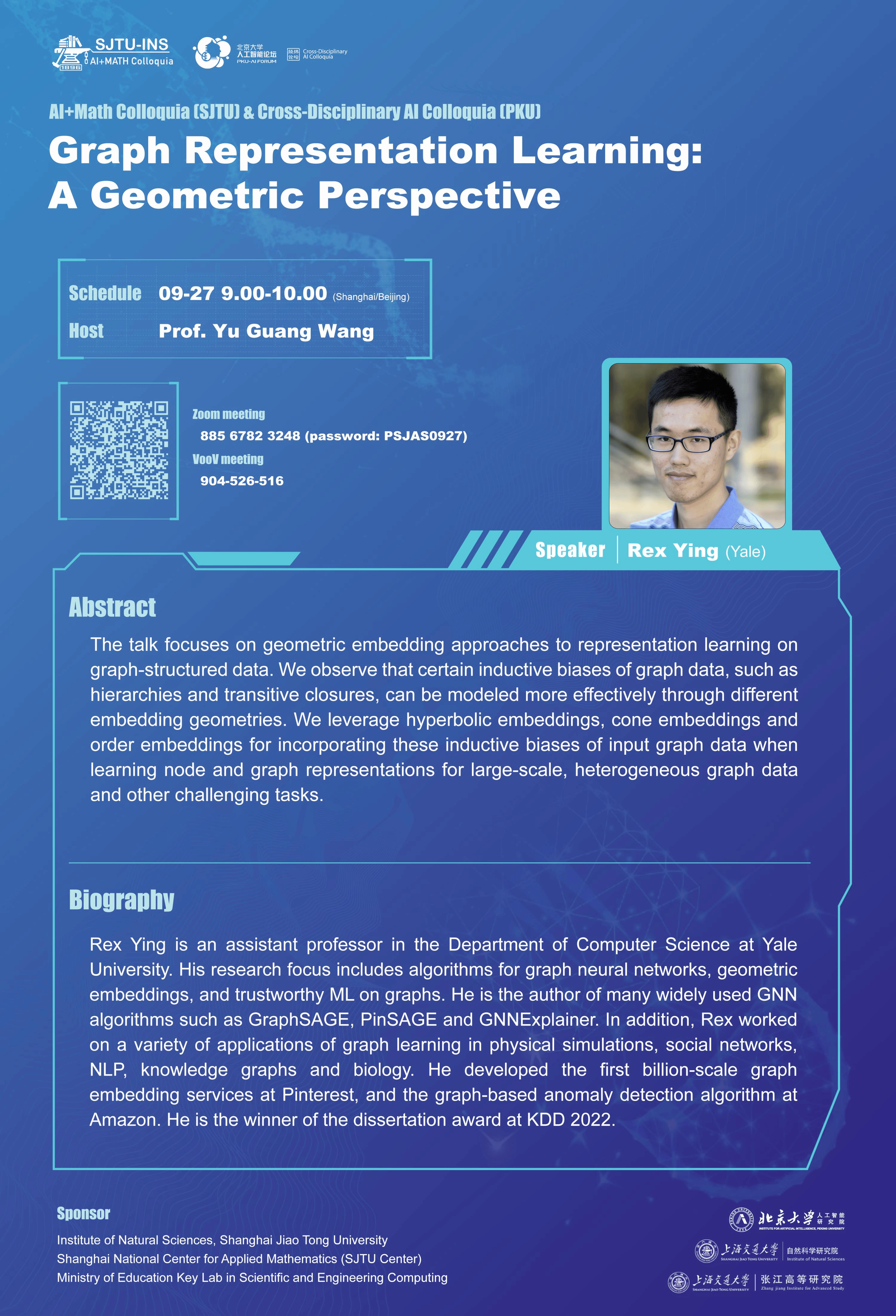
报告人:Rex Ying
时 间:2022/09/27 上午9:00
主持人:Prof. Yu Guang Wang (上海交通大学自然科学研究院)
Abstract
The talk focuses on geometric embedding approaches to representation learning on graph-structured data. We observe that certain inductive biases of graph data, such as hierarchies and transitive closures, can be modeled more effectively through different embedding geometries. We leverage hyperbolic embeddings, cone embeddings and order embeddings for incorporating these inductive biases of input graph data when learning node and graph representations for large-scale, heterogeneous graph data and other challenging tasks.
Biography
Rex Ying is an assistant professor in the Department of Computer Science at Yale University. His research focus includes algorithms for graph neural networks, geometric embeddings, and trustworthy ML on graphs. He is the author of many widely used GNN algorithms such as GraphSAGE, PinSAGE and GNNExplainer. In addition, Rex worked on a variety of applications of graph learning in physical simulations, social networks, NLP, knowledge graphs and biology. He developed the first billion-scale graph embedding services at Pinterest, and the graph-based anomaly detection algorithm at Amazon. He is the winner of the dissertation award at KDD 2022.
PSJAS(PKU-SJTU Joint AI Seminar)
PSJAS由北京大学人工智能研究院与上海交通大学自然科学研究院联合组织,是汇聚顶尖科学家的杰出系列讲座项目,旨在为图神经网络和几何深度学习方向的学术研究提供更有深度及广度的交流平台。
图神经网络和几何深度学习是近几年发展起来的深度学习方法,用于解决传统图片卷积神经网络无法有效学习的结构数据预测问题,例如药物分子设计、蛋白质结构预测、社交网络分析。2021 年,基于几何深度学习的AlphaFold 实现了对蛋白质结构的准确预测,准确率可以达到 95% 以上的实验室水平,一举破解了困扰学界长达五十年之久的“蛋白质折叠”难题。这项工作被 Science 评为 2021 年十大科学进展之最并获2023年的科学突破奖。图神经网络广泛应用于其他领域,例如它对一些数学定理的证明或发现起到了很好的作用,特别是去年发表在 Nature 上的一个用 GNN 辅助数学证明的算法,由 DeepMind 与几位科学家合作完成,引起了数学圈很大的轰动。几何深度学习作为人工智能国际前沿领域,已有诸多模型积累,然而理论基础仍很薄弱,而工业界的新挑战需要我们发展更具解释性、符合特殊应用的结构学习模型。本论坛邀请相关领域的国际知名学者、学术新星介绍几何深度学习的模型设计、数学理论和工业应用的最新进展。
PSJAS讲座项目亦纳入北京大学人工智能研究院经纬论坛系列。