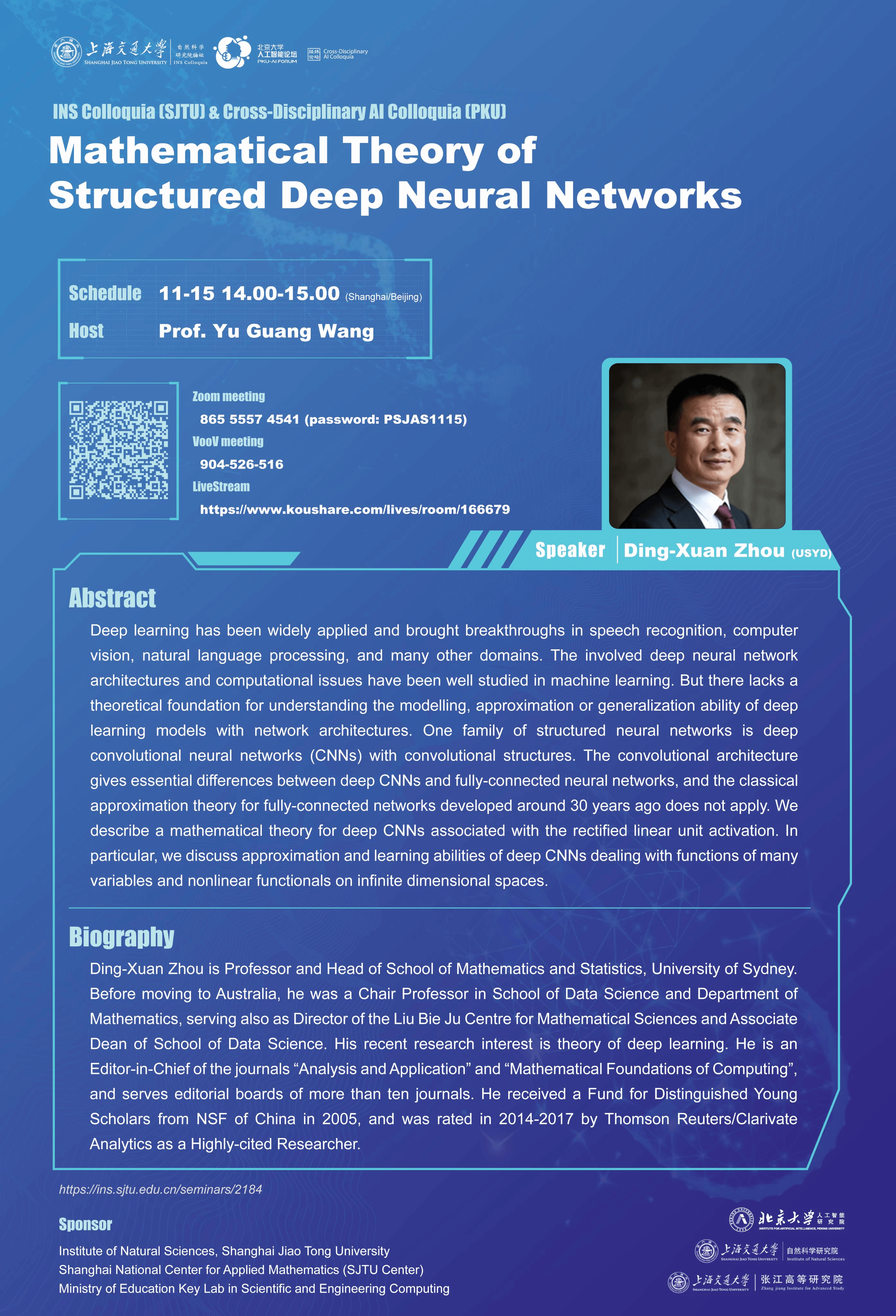
报告人:Prof. Ding-Xuan Zhou
时 间:2022/11/15 14:00 - 15:00
主持人:王宇光 教授
Title:Mathematical Theory of Structured Deep Neural Networks
Abstract:
Deep learning has been widely applied and brought breakthroughs in speech recognition, computer vision, natural language processing, and many other domains. The involved deep neural network architectures and computational issues have been well studied in machine learning. But there lacks a theoretical foundation for understanding the modelling, approximation or generalization ability of deep learning models with network architectures. One family of structured neural networks is deep convolutional neural networks (CNNs) with convolutional structures. The convolutional architecture gives essential differences between deep CNNs and fully-connected neural networks, and the classical approximation theory for fully-connected networks developed around 30 years ago does not apply. We describe a mathematical theory for deep CNNs associated with the rectified linear unit activation. In particular, we discuss approximation and learning abilities of deep CNNs dealing with functions of many variables and nonlinear functionals on infinite dimensional spaces.
Biography:
Ding-Xuan Zhou is Professor and Head of School of Mathematics and Statistics, University of Sydney. Before moving to Australia, he was a Chair Professor in School of Data Science and Department of Mathematics, serving also as Director of the Liu Bie Ju Centre for Mathematical Sciences and Associate Dean of School of Data Science. His recent research interest is theory of deep learning. He is an Editor-in-Chief of the journals “Analysis and Application” and “Mathematical Foundations of Computing”, and serves editorial boards of more than ten journals. He received a Fund for Distinguished Young Scholars from NSF of China in 2005, and was rated in 2014-2017 by Thomson Reuters/Clarivate Analytics as a Highly-cited Researcher.