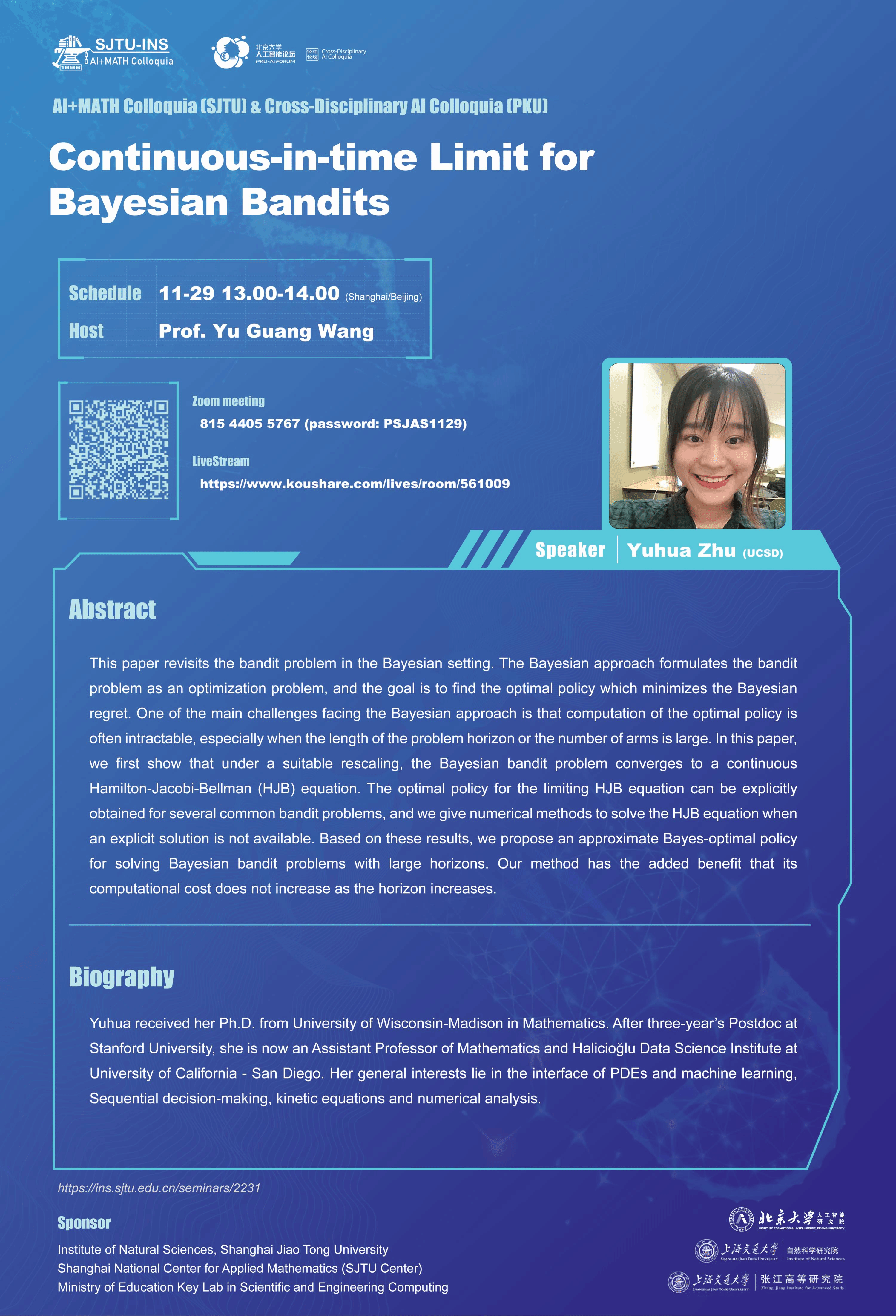
报告人:Prof. Yuhua Zhu
时 间:2022/11/29 13:00 - 14:00
主持人:王宇光 教授
Zoom:
Zoom ID: 815 4405 5767
Passcode: PSJAS1129
https://us06web.zoom.us/j/81544055767?pwd=TEZscWhySVNoS0I3QkppRUEyU1V4Zz09
Tencent Meeting:
Meeting ID: 145-282-815
https://meeting.tencent.com/dm/FH1PsuNcarbn
Live Stream:
https://www.koushare.com/lives/room/561009
Title:Continuous-in-time Limit for Bayesian Bandits
Abstract:
This paper revisits the bandit problem in the Bayesian setting. The Bayesian approach formulates the bandit problem as an optimization problem, and the goal is to find the optimal policy which minimizes the Bayesian regret. One of the main challenges facing the Bayesian approach is that computation of the optimal policy is often intractable, especially when the length of the problem horizon or the number of arms is large. In this paper, we first show that under a suitable rescaling, the Bayesian bandit problem converges to a continuous Hamilton-Jacobi-Bellman (HJB) equation. The optimal policy for the limiting HJB equation can be explicitly obtained for several common bandit problems, and we give numerical methods to solve the HJB equation when an explicit solution is not available. Based on these results, we propose an approximate Bayes-optimal policy for solving Bayesian bandit problems with large horizons. Our method has the added benefit that its computational cost does not increase as the horizon increases.
Biography:
Yuhua received her Ph.D. from University of Wisconsin-Madison in Mathematics. After three-year’s Postdoc at Stanford University, she is now an Assistant Professor of Mathematics and Halicioğlu Data Science Institute at University of California - San Diego. Her general interests lie in the interface of PDEs and machine learning, Sequential decision-making, kinetic equations and numerical analysis.