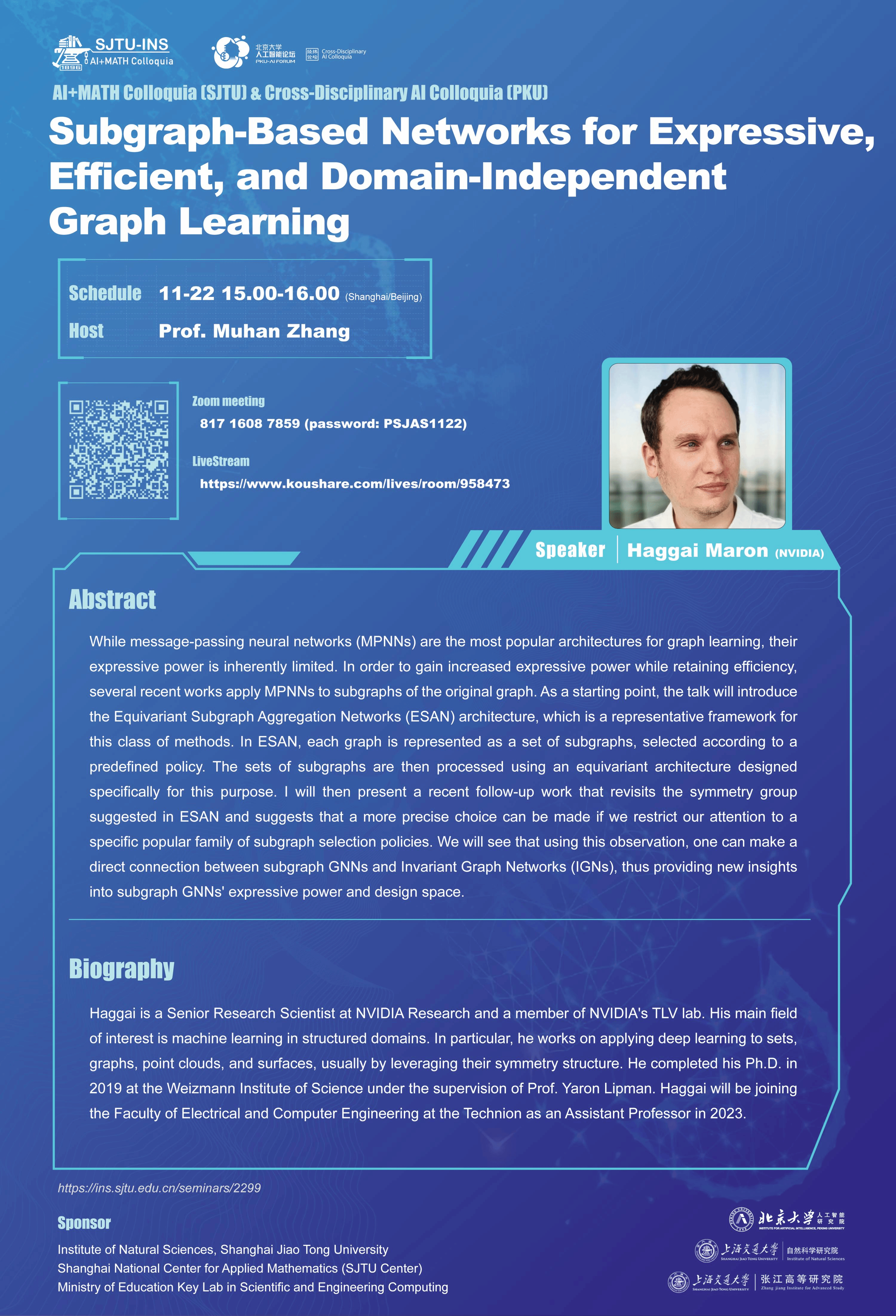
报告人:Dr. Haggai Maron
时 间:2022/11/22 15:00 - 16:00
主持人:张牧涵 助理教授
Title:Subgraph-based Networks for Expressive, Efficient, and Domain-Independent Graph Learning
Abstract:
While message-passing neural networks (MPNNs) are the most popular architectures for graph learning, their expressive power is inherently limited. In order to gain increased expressive power while retaining efficiency, several recent works apply MPNNs to subgraphs of the original graph. As a starting point, the talk will introduce the Equivariant Subgraph Aggregation Networks (ESAN) architecture, which is a representative framework for this class of methods. In ESAN, each graph is represented as a set of subgraphs, selected according to a predefined policy. The sets of subgraphs are then processed using an equivariant architecture designed specifically for this purpose. I will then present a recent follow-up work that revisits the symmetry group suggested in ESAN and suggests that a more precise choice can be made if we restrict our attention to a specific popular family of subgraph selection policies. We will see that using this observation, one can make a direct connection between subgraph GNNs and Invariant Graph Networks (IGNs), thus providing new insights into subgraph GNNs' expressive power and design space.
Biography:
Haggai is a Senior Research Scientist at NVIDIA Research and a member of NVIDIA's TLV lab. His main field of interest is machine learning in structured domains. In particular, he works on applying deep learning to sets, graphs, point clouds, and surfaces, usually by leveraging their symmetry structure. He completed his Ph.D. in 2019 at the Weizmann Institute of Science under the supervision of Prof. Yaron Lipman. Haggai will be joining the Faculty of Electrical and Computer Engineering at the Technion as an Assistant Professor in 2023.