Recently, Assistant Professor Yixin Zhu's team from the Institute for Artificial Intelligence at Peking University, in collaboration with Researcher Siyuan Huang's team from the Beijing Institute for General Artificial Intelligence, published a paper titled "Scaling Up Dynamic Human-Scene Interaction Modeling" at CVPR 2024. This research focuses on human-scene interaction motion generation, introducing innovative contributions in modeling, methodology, and training data. The authors propose a motion generation framework using an autoregressive conditional diffusion model, achieving rich, realistic, and length-unrestricted human motion generation. By introducing a local scene representation method, scene information is efficiently integrated as a condition into the diffusion model. For long-duration motion sequences, the research team proposes a simple yet effective progress identifier, enabling the autoregressive generation mechanism to fully capture the semantics of the motion. Additionally, the team released TRUMANS, the largest human-scene interaction dataset to date, containing detailed annotations of human motions and 3D scenes.
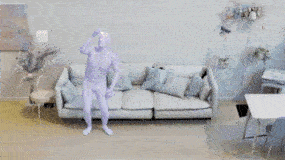
Figure 1: Using hand trajectories to control human motions that comply with scene constraints.
In daily life, people effortlessly perform various actions, such as sitting on a chair, picking up a bottle, or opening a drawer. Replicating the natural fluidity of these actions is a goal of simulation technology. Currently, simulating human limb movements has become a hot topic in fields like computer vision, computer graphics, robotics, and human-computer interaction. The core objective of human motion generation is to create natural, realistic, and diverse dynamic patterns, which have broad applications in film, gaming, augmented reality, and virtual reality. The rapid development of deep learning has significantly advanced human motion generation techniques. Breakthroughs in human modeling have made capturing motions from videos and constructing large-scale motion databases more convenient and efficient. Building upon these technological innovations, data-driven human motion generation is swiftly becoming a new focus in the research community.
Currently, research on generating human motions under specific scene and action conditions is still in its infancy, primarily due to the lack of high-quality human motion and scene interaction datasets. Existing real-world scene datasets, such as PiGraphs and PROX, still have shortcomings in human motion annotation quality. Although motion capture datasets recorded using equipment like VICON can provide high-quality motion annotations, they lack diverse human-scene interactions in 3D environments. Recently, synthetic datasets using virtual simulation technology have attracted researchers' attention due to their low cost and high adaptability.
This study introduces a new human-scene interaction dataset, TRUMANS, effectively and accurately replicating 3D synthetic scenes into physical environments that balance quality and scale. The dataset includes 15 hours of long-term human motion data, covering 100 scene configurations such as bedrooms, dining rooms, and offices. TRUMANS encompasses comprehensive daily behaviors, including navigation, object manipulation, and interactions with rigid and articulated objects. Although the scenes are synthetic, meticulous replication ensures that interactions between humans and objects can be seamlessly, naturally, and accurately reproduced.
The research proposes a method capable of generating realistic human motions under specific scene and action type conditions. A local scene perceiver is designed to recognize surrounding environmental features and generate affordance-compliant interactive motions in response. This method excels in obstacle avoidance capabilities within 3D environments—by encoding motion information, temporal information is integrated into motion segments, allowing the model to receive instructions at any time and generate corresponding motions, achieving frame-by-frame motion labels as controllable conditions. An autoregressive diffusion model technique is employed to generate continuous motions of arbitrary length.
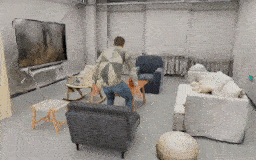
Figure 2: The TRUMANS dataset originates from meticulous motion capture and realistic rendering, allowing for diverse scene replacements.
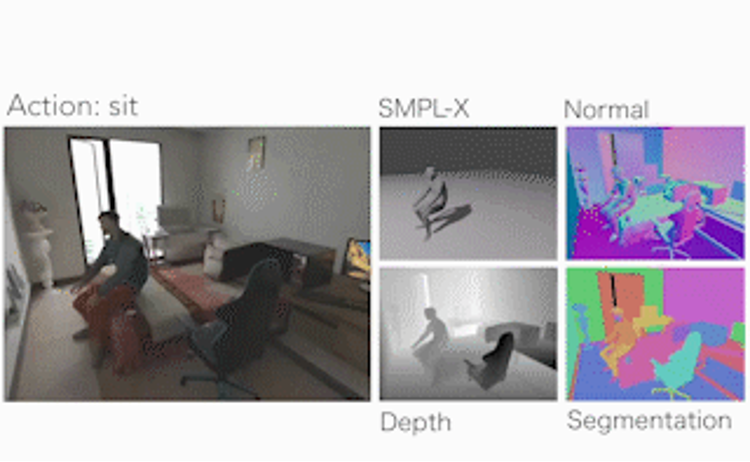
Figure 3: The TRUMANS dataset includes detailed annotations of humans, scenes, and actions.
The research objectives and methods for action generation are divided into three parts: the action generation framework based on autoregressive diffusion models, the operation of diffusion models within each cycle, and the method of incorporating scene information and action category information as conditions into the model.
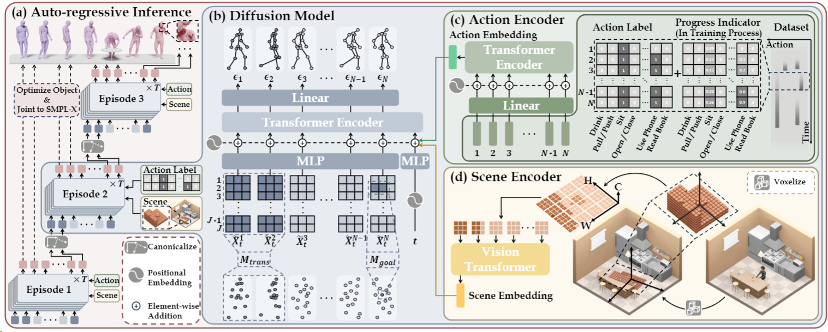
Figure 4: Method for motion generation based on an autoregressive conditional diffusion model.
This study proposes an autoregressive diffusion strategy that generates long action sequences step by step by linking each cycle's beginning and end. This approach generates controllable, natural, and diverse human body motions of arbitrary lengths. Each cycle extends the last few frames of the previous cycle, allowing the next segment to seamlessly connect with it. Fixed data on transition frames are marked with a mask, and the training process completes each segment's remaining part by filling in the frames that are not masked. Due to the use of a classifier-free training mechanism, this framework can also be applied to generate the first cycle.
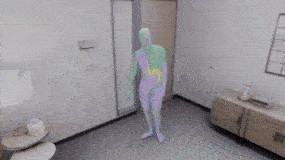
Figure 5: Diverse and scene-compliant generation results.
The local scene perceiver is used to capture local scene geometry information and condition motion generation. Specifically, given a scene, a global occupancy grid is first generated, where each cell is assigned a Boolean value indicating its reachability—1 for reachable and 0 for unreachable.
The local occupancy grid is a three-dimensional grid centered on the sub-goal of the current cycle, with a vertical range from 0 to 1.8 meters. Its orientation aligns with the yaw direction of the character's pelvis in the first frame. The values of the local occupancy grid are obtained by querying the global occupancy grid.
The proposed method utilizes a Vision Transformer (ViT) to encode voxel grids. By partitioning the local occupancy grid along the xy-plane and treating the z-axis as feature channels, tokens are constructed and input into the ViT model, using the output scene embeddings as conditions for the diffusion model. Although discretizing the scene into grids reduces the precision of human-scene interactions, this is necessary for improving training efficiency and the practicality of the method.
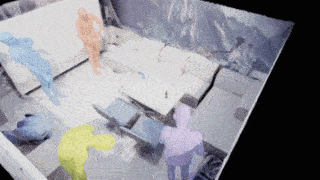
Figure 6: Dynamic objects involved in human motion generation.
The proposed method generates long-horizon actions by conditioning on action labels frame by frame. In this model, a specific action may span multiple cycles and continue across several iterations. Therefore, the model needs to understand the execution progress of an action. To support frame-by-frame action classification, a progress identifier is introduced. This identifier is a number between 0 and 1, appended to the original action label to indicate the current cycle's progress within the entire action. By incorporating this mechanism, the model can handle actions spanning multiple cycles, enhancing the semantic coherence and continuity of the generated action sequences.
The first authors of this paper are Nan Jiang, a doctoral student at the Institute for Artificial Intelligence, Peking University (supervised by Yixin Zhu), and Zhiyuan Zhang, an undergraduate student from Tsinghua University. The corresponding authors are Yixin Zhu and Siyuan Huang. Other contributors include Hongjie Li, an intern at the Institute for Artificial Intelligence, Peking University; Xiaoxuan Ma, a doctoral student at the Center on Frontiers of Computing Studies, Peking University (supervised by Yizhou Wang); Zan Wang, a doctoral student at Beijing Institute of Technology (supervised by Wei Liang); and Yixin Chen and Tengyu Liu, researchers at the Beijing Institute for General Artificial Intelligence.