Recently, an oral paper titled "X-VoE: Measuring eXplanatory Violation of Expectation in Physical Events" was presented at ICCV 2023. This research aims to build intelligent agents that understand and explain physical "magic" phenomena like humans, especially how to deal with situations where some elements are occluded or invisible. This groundbreaking research not only proposes a brand-new evaluation method with explanatory capabilities, but also demonstrates a new model that excels at handling occluded objects.
Understanding Intuitive Physics
Drawing references from the developmental process of early human intelligence, building intelligent agents that think like humans has been an important direction in AI development. However, although intuitive physics as a core knowledge of early human intelligence has received increasing attention, existing research still treats it as a prediction problem, ignoring the explanatory process of observation results. Developmental psychologists have verified humans' early cognition of intuitive physics through constructing "magic"-like violation-of-expectation experiments (VoE). They found that human participants actively explained phenomena that violated physics, and participants were surprised only when the phenomena could not be explained. This observation suggests that the explanatory process after observation is indispensable in VoE.
Violation-of-Expectation Experiments Test Physical Cognition
VoE tests cognitive abilities by observing infants' reactions to possible and impossible events. Results show that even infants react to objects that magically pass through another solid or bounce back without contact. The experimental procedure usually involves showing infants a series of strictly designed events, which either align with or violate their expectations of the world. This contrastive test design allows researchers to observe and analyze infants' reactions. Influenced by this, research teams from DeepMind, MIT, and other institutions also treat it as a testing scheme for intelligent agents' intuition of physics. However, these works only consider the prediction abilities of intelligent agents without seriously considering explanatory abilities.
Cognitive Evaluation Including Explanatory Abilities
We complete the evaluation of explanatory abilities through the three different testing schemes shown in the figure. In the basic predictive setting, when hidden factors are revealed to the model, we can simply calculate whether a video violation of physical laws, meaning prediction-only models and explanation-included models would produce the same relative surprise scores for the two examples. However, in the hypothetical setting, results could vary drastically depending on whether reasoning about hidden factors is performed: relying solely on visual perception, people may be surprised that the ball returns to the starting point instead of going through directly; but explanation-triggered participants would infer there is a blocker behind the wall, thus feeling no surprise. Since the random baseline and explanation-included model would not produce comparable different scores in these two cases, we introduce the third explanatory setting to distinguish them. These three experimental settings can identify whether an intelligent agent has explanatory abilities when dealing with VoE events.
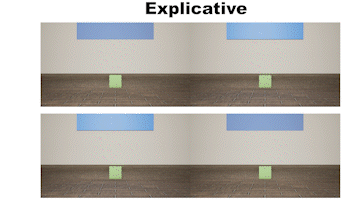
VoE Testing Dataset with Explanations
According to the three different experimental setting schemes described above (labeled as S1, S2, S3), we created four different testing scenarios covering ball collision, occlusion, object permanence, and object continuity, as shown in the figure. To test different intuitive physics laws, except for the object permanence testing scenario, each scenario is designed with three unique settings: predictive, hypothetical, and explanatory. To properly create VoE effects, we add occluding walls in front of objects in each scenario to hide some objects, and we realize different effects by changing the way the walls rise and lower. In each case, we use setting codes with the same identifiers (S1, S2, S3) to connect frames in testing videos of each setting. From start frames (first-row images) to end frames (third-row images), black connections indicate videos aligning with intuitive physics, while red connections indicate violation videos. Note that the purpose of this dataset design is mainly for testing models' understanding of intuitive physics, without limiting training data.
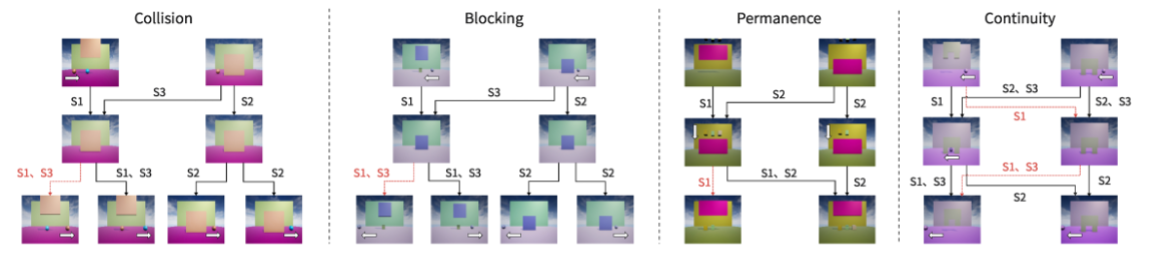
Physical Learning Model with Explanations
To introduce explanatory abilities, we add a reasoning module on top of the existing benchmark model PLATO, forming the physical learning model with explanations (XPL) shown in the figure. The proposed XPL has three components: (1) Perception module, responsible for extracting object-centric representations for downstream processing; (2) Reasoning module, responsible for inferring occluded object states from spatial and temporal contexts; (3) Dynamics module, responsible for learning physics knowledge and evaluating inferred results of occluded objects.
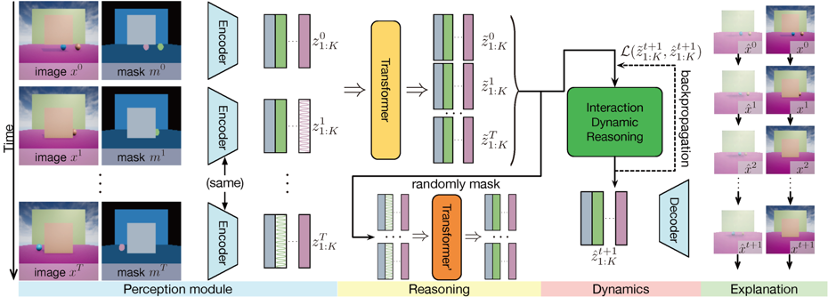
Visualizing Physical Processes Behind Occluders
A highlight of our work is the introduction of explanatory processes after observation. Here we demonstrate the physical processes behind the occluder inferred by the reasoning part of our model under the three different settings in the occlusion scenario. As shown, our model can gradually figure out there should be some other objects behind the wall, thus explaining the observation results and judging whether intuitive physics is violated based on the explained physical processes.
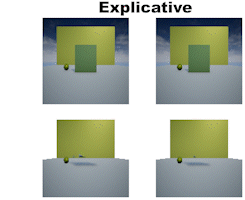
Quantitative Results and Metrics
We analyze the model accuracy from overall and comparative perspectives, comparing PLATO and PhyDNet models. In the figures, ideal values are marked by the red line positions.
Overall accuracy: From an overall perspective, the model should be able to make an overall judgment on whether intuitive physics is violated. We compare all videos of violation and non-violation under the same scenario across all settings to obtain the overall evaluation metric. As shown, XPL and PLATO demonstrate better performance in all testing scenarios but still have an obvious gap from perfect performance. XPL significantly outperforms PLATO in collision, occlusion, and permanence, but falls behind in continuity. We also compare different dynamics modules of XPL (with and without residual connections), finding that adding residuals improves model performance.
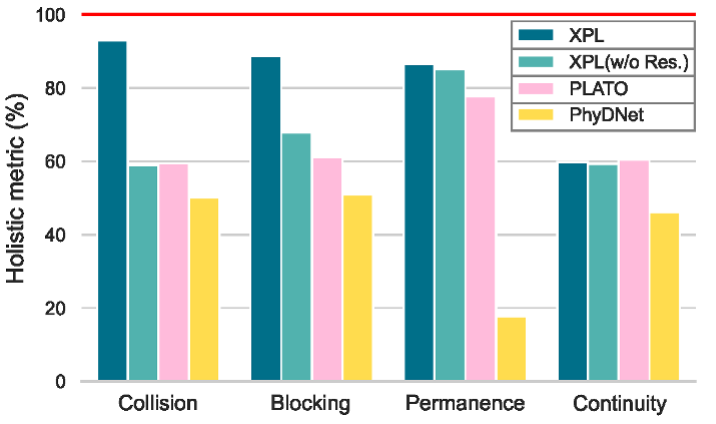
Comparative accuracy: From a comparative view, the model should be able to judge which of two contrasting videos violates intuitive physics more. We compare results of two videos in the same setting under the same scenario to obtain the comparative evaluation metric. In the figure, the ideal value for the hypothetical environment is set to 50% instead of 100% since the two videos should have relatively equal scores. Results in the predictive environment indicate current AI systems can achieve good evaluation results for testing tasks relying solely on predictive abilities. In the hypothetical environment, both the random human participants baseline and ideal human participants have 50% accuracy. This is exactly why this problem attracts psychologists' attention, as experiments on infants show no violation of expectation, which could mean they lack physical common sense, but could also imply explanatory abilities. The explanatory setting provides information behind the occluder near the end of the videos, turning possible scenarios in the hypothetical setting into impossible ones. This change reflects the influence of explanatory abilities, and our model demonstrates the transition of results from hypothetical to explanatory settings in the collision and occlusion scenarios, especially in the collision scenario where the transition approaches 90%.
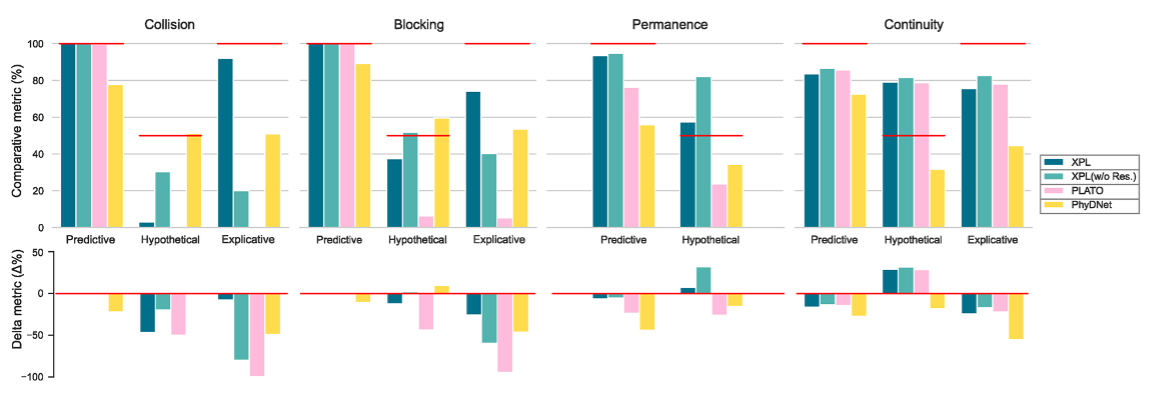
In this work, the researchers introduce that explanatory ability should be an important part of evaluating intuitive physics. The researchers provide a novel explanation-based violation-of-expectation video dataset with three unique settings: predictive, hypothetical and explanatory. While the predictive setting is consistent with traditional violation-of-expectation video testing, the other two settings focus on evaluating models' explanatory abilities. The proposed model combines reasoning and explanatory processes to handle occluded objects, providing higher performance in the dataset settings. Experiments show the explanation-included model excels in scenarios requiring explicit explanation of occluded objects, surpassing other methods. Notably, reasoning about physics behind occluders provides visualizable explanations for occlusion events, highlighting its ability to reason about hidden factors. Bo Dai is the first author, and Linge Wang, Baoxiong Jia, Zhang Zeyu, Song-Chun Zhu, Chi Zhang and Yixin Zhu are co-authors of the paper.
Paper Link: https://yzhu.io/publication/intuitive2023iccv/